Evaluating complex models in ecology when autocorrelation is present
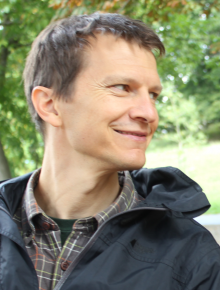
Volker Bahn
Wright State University, Dayton, USA volker.bahn@wright.edu
(Talk in English)
Ecological research often produces messy data that necessitates flexible and complex models. However, complex models can overfit data, leading to a decoupling between goodness-of-fit and predictive power of the model, making goodness-of-fit an unsuitable evaluation measure. The alternative is to hold out test data for evaluation. However, when autocorrelation is present in model residuals, training and test can lack independence and model evaluation will be overly optimistic. I discuss strategies for decoupling training and test data in the context of species distribution models. While these strategies can reduce the underestimation of model error, they can introduce over-estimation of error by introducing extrapolation in variable space. This problem can be addressed by explicitly testing for analog conditions between training and test data, leading to realistic error estimates.
Recent publications:
Lavretsky, P., J. L. Peters, K. Winker, V. Bahn, I. Kulikova, Y. N. Zhuravlev, R. E. Wilson, C. Barger, K. Gurney, and K. G. McCracken. 2016. Becoming pure: identifying generational classes of admixed individuals within lesser and greater scaup populations. Molecular Ecology 25:661-674.
Bahn, V. and B.J. McGill. 2013. Assessing the predictive power of distribution models. Oikos 122: 321–331.
Bahn, V. 2008. Failure to find the relationship between dispersal and spatial autocorrelation in species distributions. Journal of Negative Results in Ecology and Evolution 5(1):1-13.
Contact: Xavier Morin; xavier.morin@cefe.cnrs.fr
Contact du Comité SEEM: seem@services.cnrs.fr. Contact du Labex CEMEB: gestion.cemeb@univ-montp2.fr, www.labex-cemeb.org.